Leadership explainer: k-anonymity, l-diversity and t-closeness
There are many anonymisation techniques that companies can choose depending upon the nature of their business, business sector, jurisdiction, risk, complexity of processing and maturity/internal competences to mention a few factors. All of these techniques are complex.
DE-IDENTIFICATION TECHNIQUESEXPLAINER
Tim Clements
8/8/20243 min read
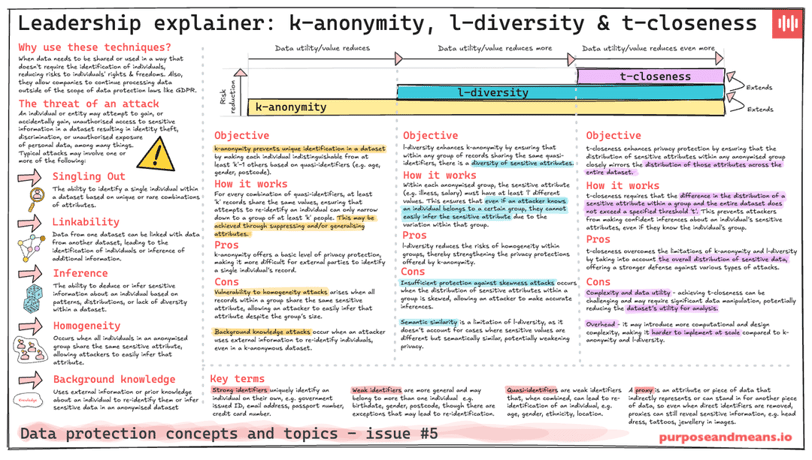
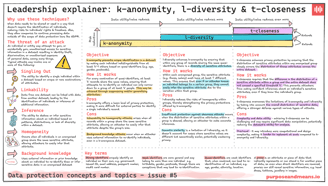
In order to begin to explain the techniques to other colleagues, including senior leadership we need to simplify and present the topics in simple terms. In this blog post I explore three techniques that are closely related: k-anonymity, l-diversity and t-closeness. In future posts I'll cover other techniques, also in simple terms.
This one-page explainer is a useful reference if you need to provide your steering committee or board with an overview of some of the more technical aspects of your data protection work.
Why use these techniques?
When data needs to be shared or used in a way that doesn't require the identification of individuals, reducing risks to individuals' rights & freedoms. Also, they allow companies to continue processing data outside of the scope of data protection laws like GDPR.
The threat of an attack
An individual or entity may attempt to gain, or accidentally gain, unauthorised access to sensitive information in a dataset resulting in identity theft, discrimination, or unauthorised exposure of personal data, among many things.
Typical attacks may involve one or more of the following:
Singling Out
The ability to identify a single individual within a dataset based on unique or rare combinations of attributes.Linkability
Data from one dataset can be linked with data from another dataset, leading to the identification of individuals or inference of additional information.Inference
The ability to deduce or infer sensitive information about an individual based on patterns, distributions, or lack of diversity within a dataset.Homogeneity
Occurs when all individuals in an anonymised group share the same sensitive attribute, allowing attackers to easily infer that attribute.Background knowledge
Uses external information or prior knowledge about an individual to re-identify them or infer sensitive data in an anonymised dataset
Key terms
Strong identifiers uniquely identify an individual on their own, e.g. government issued ID, email address, passport number, credit card number.
Weak identifiers are more general and may belong to more than one individual e.g. birthdate, gender, postcode, though there are exceptions that may lead to re-identification.
Quasi identifiers are weak identifiers that, when combined, can lead to re-identification of an individual, e.g. age, gender, ethnicity, location.
A proxy is an attribute or piece of data that indirectly represents or can stand in for another piece of data, so even when direct identifiers are removed, proxies can still reveal sensitive information, e.g. head dress, tattoos, jewellery in images.
k-anonymity
Objective
k-anonymity prevents unique identification in a dataset by making each individual indistinguishable from at least 'k'−1 others based on quasi-identifiers (e.g. age, gender, postcode).How it works
For every combination of quasi-identifiers, at least 'k' records share the same values, ensuring that attempts to re-identify an individual can only narrow down to a group of at least 'k' people. This may be achieved through suppressing and/or generalising attributes.Pros
k-anonymity offers a basic level of privacy protection, making it more difficult for external parties to identify a single individual’s record.Cons
Vulnerability to homogeneity attacks arises when all records within a group share the same sensitive attribute, allowing an attacker to easily infer that attribute despite the group’s size.
Background knowledge attacks occur when an attacker uses external information to re-identify individuals, even in a k-anonymous dataset.
l-diversity
Builds upon k-anonymity.
Objective
l-diversity enhances k-anonymity by ensuring that within any group of records sharing the same quasi-identifiers, there is a diversity of sensitive attributes.How it works
Within each anonymised group, the sensitive attribute (e.g. illness, salary) must have at least 'l' different values. This ensures that even if an attacker knows an individual belongs to a certain group, they cannot easily infer the sensitive attribute due to the variation within that group.Pros
l-diversity reduces the risks of homogeneity within groups, thereby strengthening the privacy protections offered by k-anonymity.Cons
Insufficient protection against skewness attacks occurs when the distribution of sensitive attributes within a group is skewed, allowing an attacker to make accurate inferences.
Semantic similarity is a limitation of l-diversity, as it doesn’t account for cases where sensitive values are different but semantically similar, potentially weakening privacy.
t-closeness
Builds upon l-diversity.
Objective
t-closeness enhances privacy protection by ensuring that the distribution of sensitive attributes within any anonymised group closely mirrors the distribution of those attributes across the entire dataset.How it works
t-closeness requires that the difference in the distribution of a sensitive attribute within a group and the entire dataset does not exceed a specified threshold 't'. This prevents attackers from making confident inferences about an individual’s sensitive attributes, even if they know the individual's group.Pros
t-closeness overcomes the limitations of k-anonymity and l-diversity by taking into account the overall distribution of sensitive data, offering a stronger defense against various types of attacks. l-diversity reduces the risks of homogeneity within groups, thereby strengthening the privacy protections offered by k-anonymity.Cons
Complexity and data utility - achieving t-closeness can be challenging and may require significant data manipulation, potentially reducing the dataset’s utility for analysis.
Overhead - it may introduce more computational and design complexity, making it harder to implement at scale compared to k-anonymity and l-diversity.
Purpose and Means
Purpose and Means believes the business world is better when companies establish trust through impeccable governance.
BaseD in Copenhagen, OPerating Globally
tc@purposeandmeans.io
+45 6113 6106
© 2025. All rights reserved.